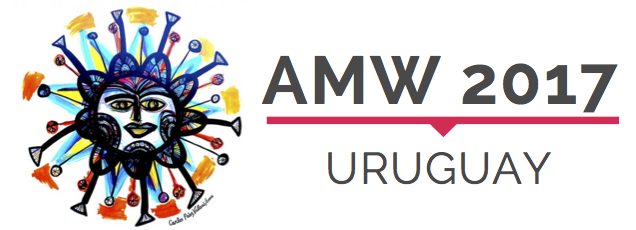
Keynotes
Keynote Speakers
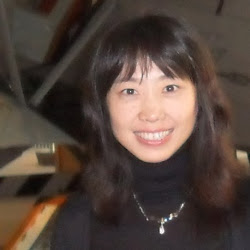
Leave No Valuable Data Behind: the Crazy Ideas and the Business
Xin Luna Dong.
Abstract:
With the mission "leave no valuable data behind", we developed techniques for knowledge fusion to guarantee the correctness of the knowledge. This talk starts with describing a few crazy ideas we have tested. The first, known as "Knowledge Vault", used 15 extractors to automatically extract knowledge from 1B+ Webpages, obtaining 3B+ distinct (subject, predicate, object) knowledge triples and predicting well-calibrated probabilities for extracted triples. The second, known as "Knowledge-Based Trust", estimated the trustworthiness of 119M webpages and 5.6M websites based on the correctness of their factual information. We then present how we bring the ideas to business in filling the gap between the knowledge in existing knowledge bases and the knowledge in the world.
Speaker Bio
Xin Luna Dong is a Principal Scientist at Amazon, leading the efforts of constructing Amazon Product Knowledge Graph. She was one of the major contributors to the Knowledge Vault project, and has led the Knowledge-based Trust project, which is called the "Google Truth Machine" by Washington's Post. She has co-authored book "Big Data Integration", published 65+ papers in top conferences and journals and given 20+ keynotes/invited-talks/tutorials. She got the VLDB Early Career Research Contribution Award for advancing the state of the art of knowledge fusion, and got the Best Demo award in Sigmod 2005. She is the PC co-chair for Sigmod 2018 and WAIM 2015, and serves as an area chair for Sigmod 2017, Sigmod 2015, ICDE 2013, and CIKM 2011.
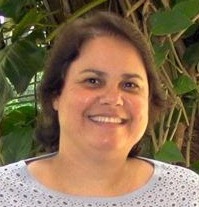
Querying on Complex Databases by Content and Context – Challenges and Real Applications
Agma J. M. Traina
Abstract:
The amount and complexity of data generated and managed in nowadays systems, such as images, videos, and time series among others, bring several challenges to the data management developers in order to comply with the expectation of the users and data owners. Not only the majority of the applications demand searching complex data through queries considering several different aspects on the same data, but also getting the answers in a timely manner. Content-based similarity retrieval enables performing queries and analyses using the required features automatically extracted from the data without user intervention.
In this talk we will discuss the challenges posed to the database and related communities in order to provide techniques and tools to overcome the precision and time concerns regarding similarity queries over complex data. Examples and results obtained with two decades long experience over real applications will be presented and discussed.
Speaker Bio
Agma J. M. Traina is a Professor with the Computer Science Department of the Mathematics and Computer Science Institute at the University of São Paulo at São Carlos. She received her PhD in Computational Physics from the University of São Paulo at São Carlos, Brazil, as well as her BSc and MSc in Computer Science. She spent two years as a visiting scholar at Carnegie Mellon University, where she worked with multimedia databases and selectivity estimation. Agma's research interests ranges from complex data indexing and retrieval by content, similarity queries to data visualization and visual data mining. She has focused her research on medical applications supported by image processing techniques, and more recently on climate/agriculture and remote sensing data. Over the years, she has supervised over 40 Graduate students in these areas, and published more than 250 papers in journals and conferences, as well as several awards. Agma is a member of the Brazilian Computer Society, SIAM, ACM and IEEE Computer Society.
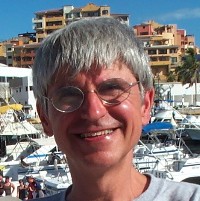
Shannon-type Inequalities for Optimal Query Processing
Dan Suciu, University of Washington.
We consider the problem of computing a conjunctive query over an input database with known statistics, with a goal of designing "optimal" algorithms. The statistics include the cardinalities of the base relations, and upper bounds on the degrees of some attributes of some relations. For example a functional dependency is a special case of a degree constraint where the degree bound is 1. We describe query evaluation algorithms using Shannon-type information-theoretic inequalities. For a full conjunctive queries, the information-theoretic inequality gives an upper bound on the size of the output of the query; the AGM bound on the output of the query is the special case of statistics restricted to cardinalities. For general conjunctive queries, the information-theoretic inequality give the (generalization of the) sub-modular width of the query. We describe algorithms that match the bounds of these Shannon-type inequalities.
Speaker Bio
Dan Suciu is a Professor in Computer Science at the University of Washington. He received his Ph.D. from the University of Pennsylvania in 1995, was a principal member of the technical staff at AT&T Labs and joined the University of Washington in 2000. Suciu is conducting research in data management, with an emphasis on topics related to Big Data and data sharing, such as probabilistic data, data pricing, parallel data processing, data security. He is a co-author of two books Data on the Web: from Relations to Semistructured Data and XML, 1999, and Probabilistic Databases, 2011. He is a Fellow of the ACM, holds twelve US patents, received the best paper award in SIGMOD 2000 and ICDT 2013, the ACM PODS Alberto Mendelzon Test of Time Award in 2010 and in 2012, the 10 Year Most Influential Paper Award in ICDE 2013, the VLDB Ten Year Best Paper Award in 2014, and is a recipient of the NSF Career Award and of an Alfred P. Sloan Fellowship. Suciu serves on the VLDB Board of Trustees, and is an associate editor for the Journal of the ACM, VLDB Journal, ACM TWEB, and Information Systems and is a past associate editor for ACM TODS and ACM TOIS. Suciu's PhD students Gerome Miklau, Christopher Re and Paris Koutris received the ACM SIGMOD Best Dissertation Award in 2006, 2010, and 2016 respectively, and Nilesh Dalvi was a runner up in 2008.
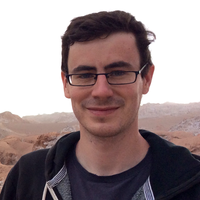
Linked Data: Turning Practice into Theory
Aidan Hogan
Now over a decade old, Linked Data has seen plenty of practice but not a lot of theory. Linked Data inherits some of its theory from its big brother the Semantic Web, who inherited much of its theory, in turn, from Knowledge Representation. Some of that KR theory has had impact on Linked Data in practice; some has not. More recently, Linked Data has also inherited some theoretical results from its wealthy uncle, the Database. Some of that theory has had direct impact on Linked Data in practice; some has not. This perhaps raises a number of questions. First: does Linked Data need (more) theory, and if so, for what? Second: what can the theory from KR, databases, etc., actually predict about the practice of Linked Data (if anything)? Third: why must Linked Data be resigned, in theoretical terms, to inheriting theory? Or, rephrasing: can Linked Data have any interesting theoretical questions of its own that are not trivially answered by stitching up the sleeves of the theory from elsewhere? In this talk, I will first give an overview of Linked Data in terms of its inception, motivation, successes and failures thus far. I will then give my own (limited) perspective on the previous questions in terms of the relationship between the theory and practice of Linked Data (and perhaps as a tenuous generalisation, to other areas of CS). The goal of the talk is then to try to explore what opportunities might exist for better bridging theory and practice in future, particularly in the context of Linked Data.
Speaker Bio
Aidan Hogan is an Assistant Professor in the Department of Computer Science (DCC) at the Universidad de Chile and an Associate Researcher of the Chilean Centre for Semantic Web Research. His main interests lie in the area of the Semantic Web and Linked Data, where he has worked on a variety of topics relating to the management of large, decentralised, diverse, dynamic and often dirty corpora of structured data (as are native to the Web). He has won various awards at international research events, including a best evaluation paper (ISWC), best poster (ESWC), and several awards for reviewing (SWJ, EKAW, ISWCx3, WedDB); he has also picked up a couple of other best paper nominations (including at ISWC and WWW). He serves on the editorial boards of the Semantic Web Journal and the Journal of Web Semantics. He has given several invited lectures at summer schools and has presented a variety of tutorials at conferences (though this will be his first real keynote).